mixOmics is collaborative project between Australia (Melbourne), France (Toulouse), and Canada (Vancouver). We have a core team, key contributors and collaborate with many researchers around the world – it could be you too if you wish to be involved: we host many visitors with computational, statistical and biological backgrounds!
The history of mixOmics
The project started at the Institut de Mathématiques de Toulouse, Université Paul Sabatier, Toulouse, France. The code was packaged in Australia, at the University of Queensland, Brisbane (2009 – 2016) and at the University of Melbourne, Australia (from 2017). We focus on the development of computational and statistical methods for biological data analysis and integration and their implementation in mixOmics.
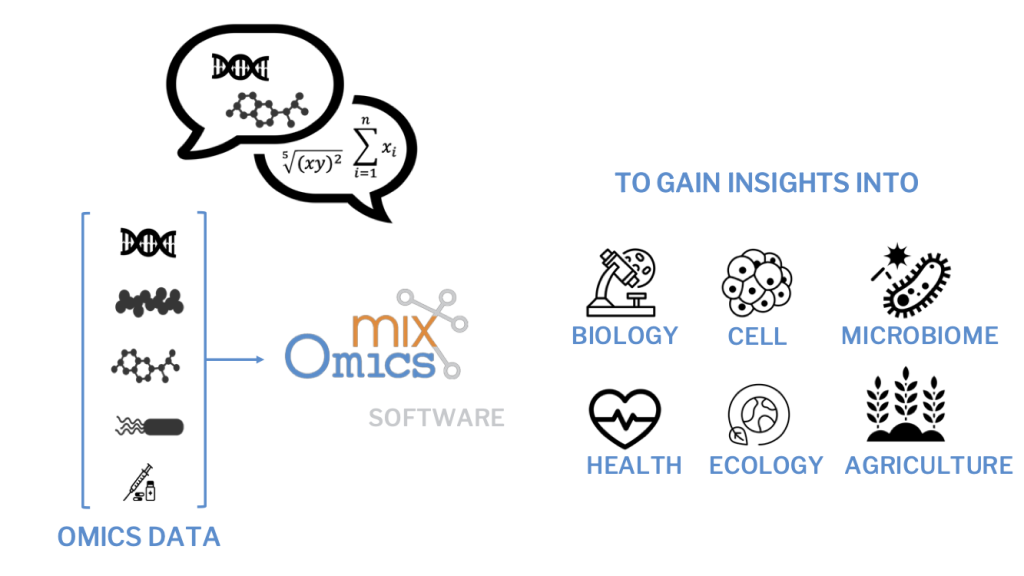
Our main milestones
- 2008: Initial Development. The foundational work on sparse Partial Least Squares (sPLS) and regularised CCA (rcc) methods began by Dr Kim-Anh Lê Cao and Dr Ignacio Gonzalez, laying the groundwork for the development of mixOmics.
- 2010: First Release on CRAN. mixOmics was officially launched on the Comprehensive R Archive Network (CRAN), providing a platform for users to access Principal Component Analysis (PCA), sPLS and rCCA for multi-omics integration and exploration.
- 2011 – 2014: Introduction of new methods. The package incorporated advanced multivariate methods for single omics (sparse PLS-Discriminant Analysis) and multi-omics (sparse Generalised CCA and PLS) and network visualisations.
- 2012: Creation of the mixOmics.org website which serves as a hub for resources, including detailed tutorials, case studies, workflows, and documentation.
- 2014: Development of workshop material to run face-to-face workshops, engaging with a growing community of users, and incorporating feedback for further development.
- 2017 – 2019: Addition of MINT and DIABLO frameworks. DIABLO (Data Integration Analysis for Biomarker discovery using Latent cOmponents) allows for simultaneous integration and feature selection across multiple data types in a classification framework; MINT allows for simultaneous integration and robust feature selection across multiple independent studies
- 2017: First release on Bioconductor to empower computational and molecular biologists, which aligns with bioC vision and provide in-depth vignette
- 2019: Creation of the discussion forum to enable regular support to our users and gather feedback
- 2021: Publication of the mixOmics handbook to complete our set of resources
- 2022: Development of online workshops to engage with a growing community of international users
- Ongoing: Regular Updates and Methodological Advances. mixOmics continues to evolve with the continuous development of cutting-edge statistical methods for new omics data types of biological questions in modern biological research.