Lê Cao team and collaborators from University of British Columbia (Vancouver, Canada) have published their first method to integrate multiple omics data from the same set of biospecimens or individuals (e.g. transcriptomics, proteomics). Their method adopts a systems biology holistic approach by statistically integrating data from multiple biological compartments. Such approach provides improved biological insights compared with traditional single omics analyses, as it allows to take into account interactions between omics layers and extract multi-omics molecular networks.
DIABLO is a multivariate dimension reduction method and is hypothesis-free. The method constructs combinations of variables (e.g. cytokines, transcripts, proteins, metabolites) that are maximally correlated across data types to identify a minimal subset of markers – a multi-omics signature. This signature can highlight novel findings but is also the starting point to network modelling.
More information about DIABLO, implemented in the mixOmics R package: Amrit Singh, Casey P Shannon, Benoît Gautier, Florian Rohart, Michaël Vacher, Scott J Tebbutt and Kim-Anh Lê Cao (2019) DIABLO: an integrative approach for identifying key molecular drivers from multi-omics assays, Bioinformatics. You can also find some technical information in the mixOmics paper (particularly in the Supp!) and also in our tutorials here.
While the computational researchers where busy developing their method, they also analysed the data from the #SmallBig study (small sample, big data) with the EPIC (Expanded Program on Immunization) Consortium. EPIC comprises researchers from the Boston Children’s Hospital, University of British Columbia, Medical Research Council Unit The Gambia, Université libre de Bruxelles, Telethon Kids Institute and University of Western Australia, the Papua New Guinea Institute for Medical Research, to answer the question: What can less than 1mL of blood tell us about a newborn’s health?
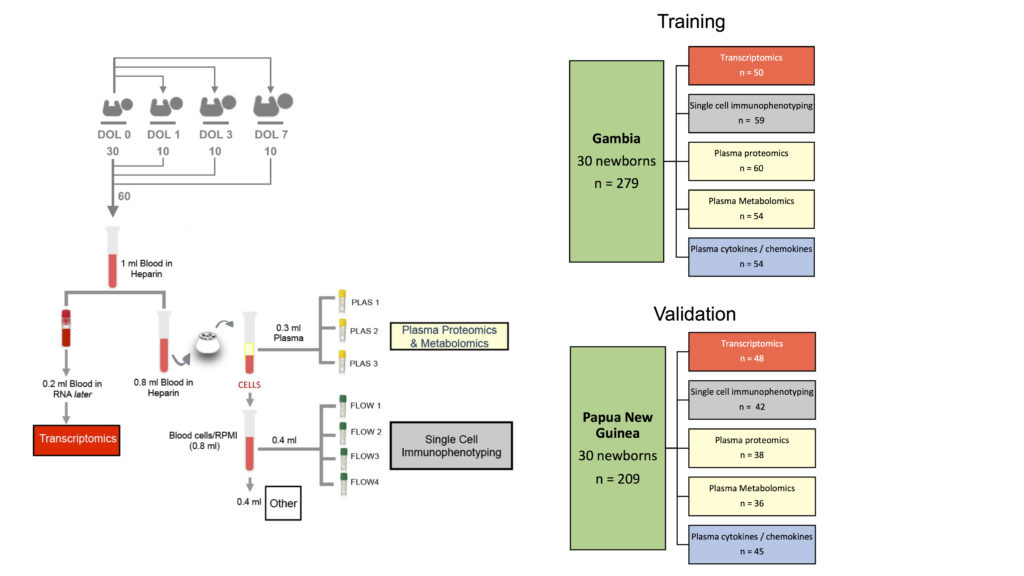
In this study recently published in Nature Communications, the team has developed a technique to collect extremely small volumes of blood samples (< 1mL) to comprehensively characterise how biological molecules evolve in newborns. Using cutting-edge computational and statistical methods including DIABLO, they show that to the contrary to biology in adults that has a relatively steady-state, the first week of human life is highly dynamic and undergoes dramatic changes. Their results were consistently observed in vastly different areas of the world, West Africa (The Gambia) and Australasian (Papua New Guinea) and suggest a purposeful rather than random developmental path.
More information about the SmallBig study: Amy H. Lee, Casey P. Shannon, […]Tobias R. Kollmann (2019). Dynamic molecular changes during the first week of human life follow a robust developmental trajectory Nature Communications volume 10, Article number: 1092.
If you are interested in the potential of DIABLO to integrate microbiome and omics from the host, here is another study we published. We integrated the microbiome, proteome and meta-proteomics in T1D individuals.
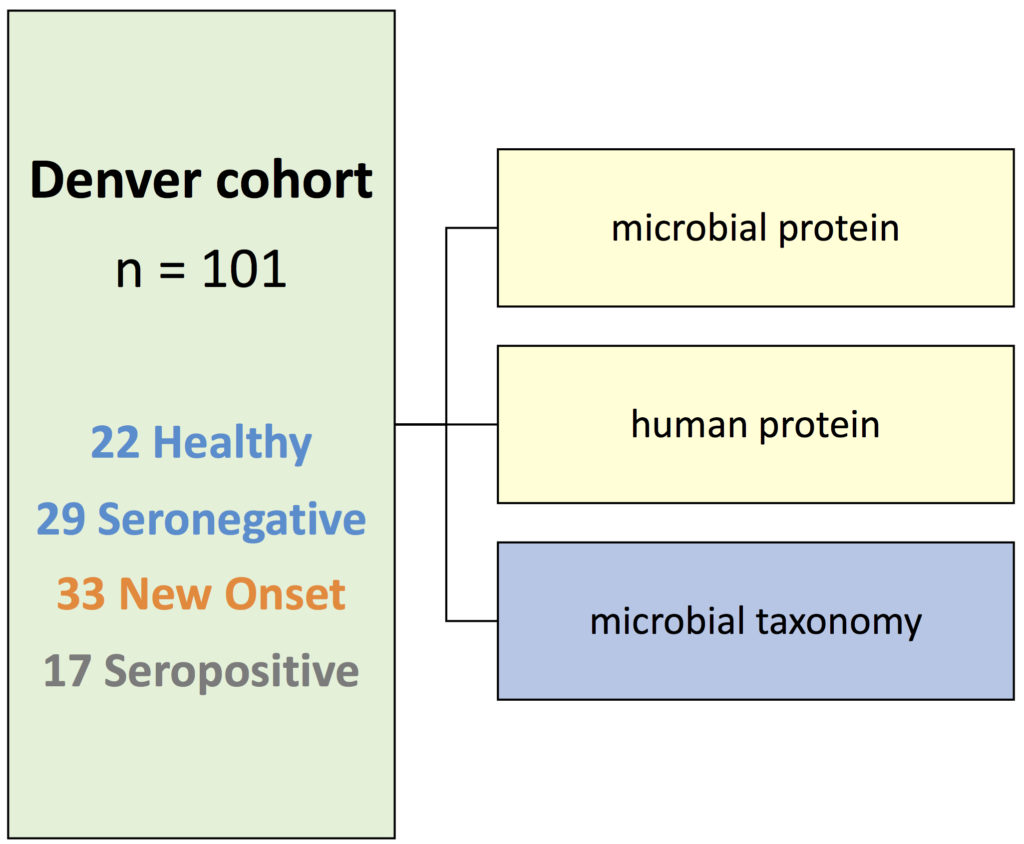
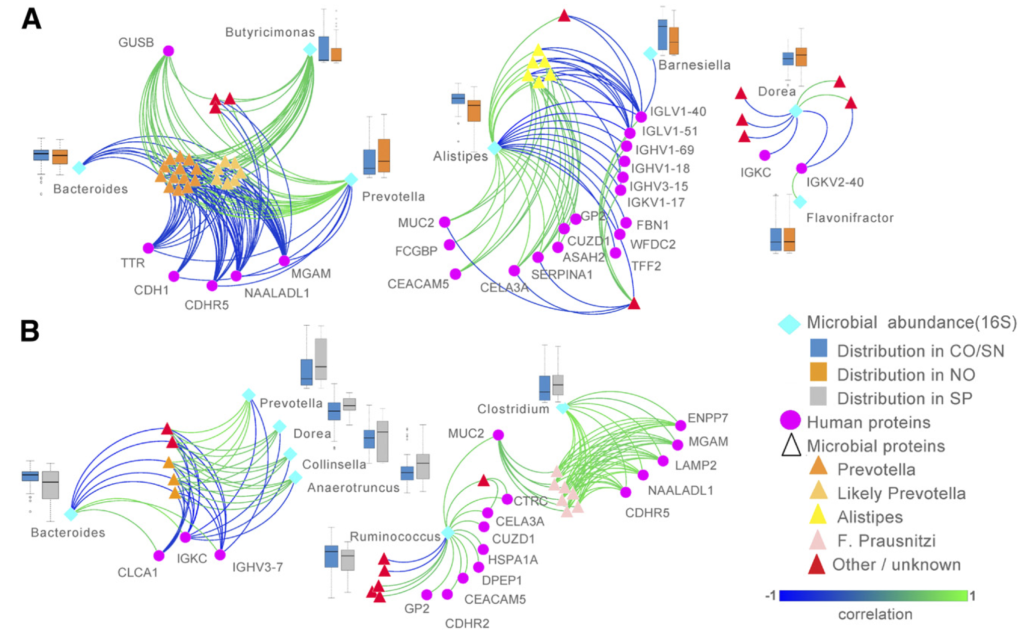
More details about the study: Gavin PG, […], and Hamilton-Williams EE (2018). Intestinal metaproteomics reveals host-microbiota interactions in subjects at risk for type 1 diabetes Diabetes care 41: 10. We used DIABLO to integrate microbiome, proteomics and meta-proteomics.