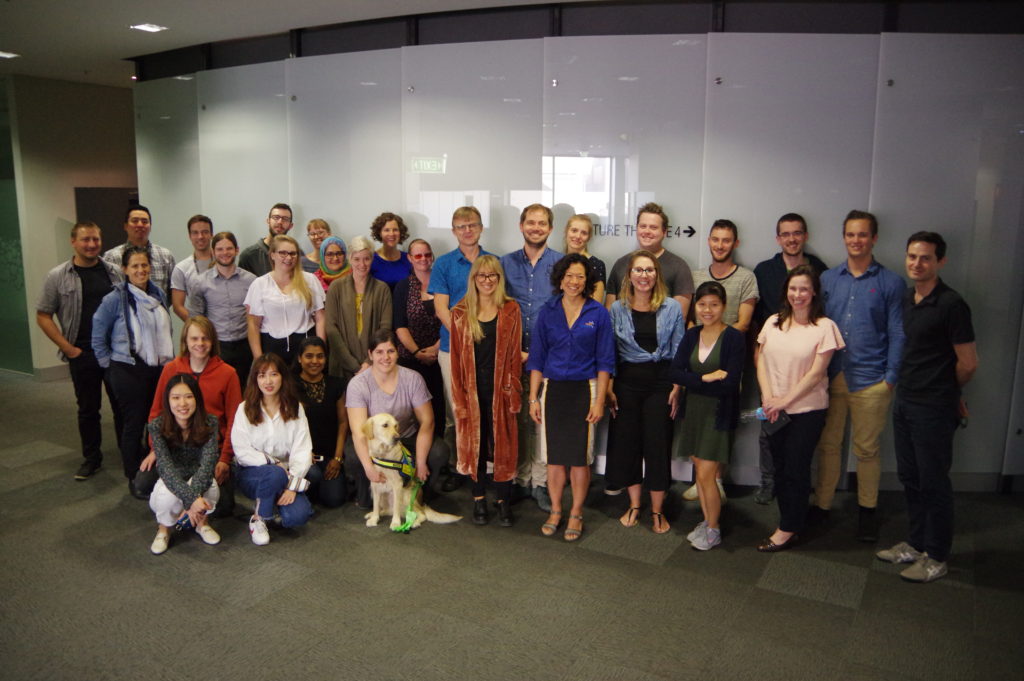
Feedback from the workshop: This time we included several new case studies specifically focused on microbiome applications. We presented new material, including the problem of compositional data, how to detect and assess existing methods for batch effects (Ms Yiwen (Eva) Wang, PhD student) and our first timeOmics pipeline (Dr Olivier Chapleur).
‘I was especially pleased with the pace of the workshop. There was time to ask questions during lectures and practice. The pracs were designed to be relevant to our actual research questions.’
The event was sponsored by AFRAN and we obtained 50% bursaries from EMRI UoM for 5 PhD students.
‘Good contextualisation of methods before application of them, lots of depth on the background to methods which was important even when concepts were very complex. ‘I think the case studies were really helpful. The R code is written in such a clear and digestible way that it was easy to apply to my own data’
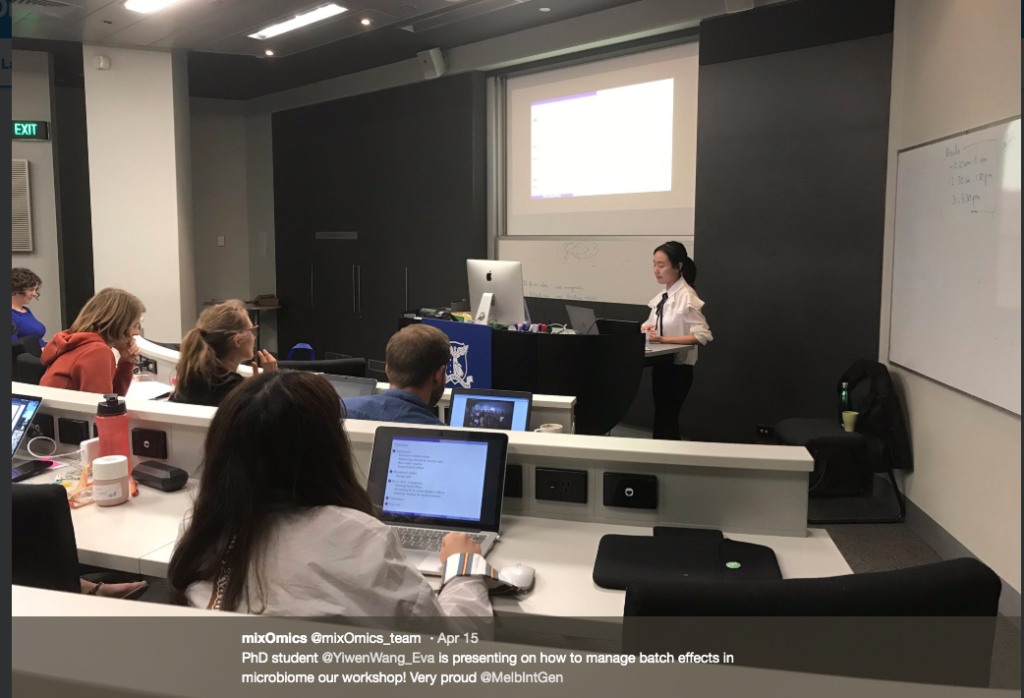
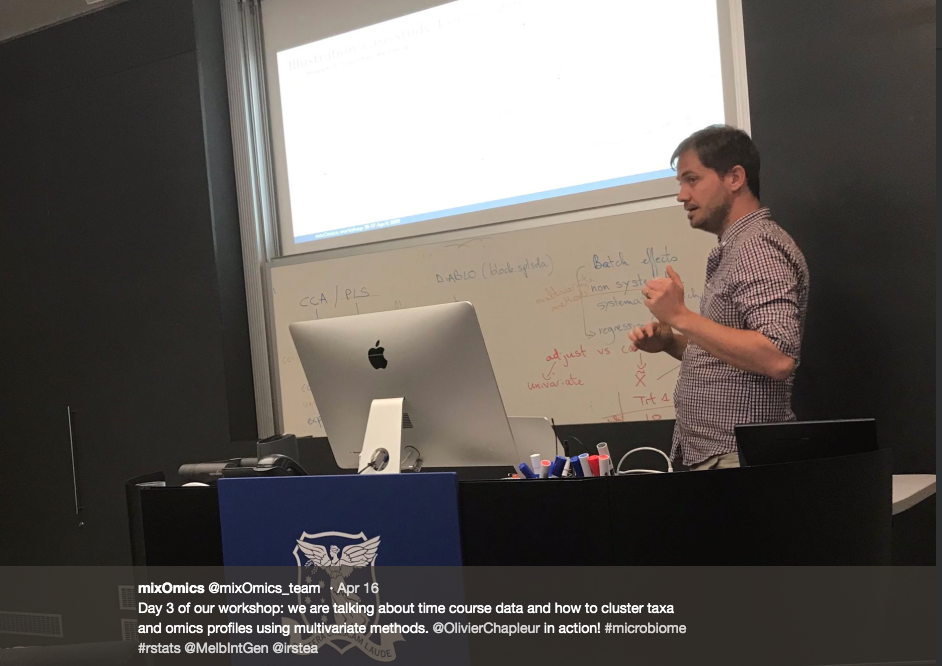
‘The pace and depth was good. All topics covered were highly relevant, and techniques were directly applicable. The ‘mood’ of the workshop was very friendly.’
Complex microbial networks have a central role in the provision and regulation of ecosystems. Multiple microbial biotechnology applications are contributing to global efforts to achieve sustainability – through purification of wastewater, waste valorisation, bioenergy production, or to understand the role of microbiome in human disease and healthy states.
Statistical analysis of microbiome data is challenging due to the inherent characteristics of the data, such as high sparsity and compositional structure. Our workshop will introduce major concepts including multivariate dimension methods developed in mixOmics. Our methods make no distributional assumptions, are highly flexible for unsupervised (exploratory), supervised (classification) and integration analyses.
This hands-on course will cover basic processing and inherent characteristics of microbiome data (compositionality, batch effects), various analytical frameworks ranging from data exploration, selection of microbial markers, integration with other omics datasets and introduction to time-course analysis. Each methodology introduced in the workshop will be illustrated on real biological studies. The third day is ‘BYO data’ day where you can reinforce your learnings on your own study!
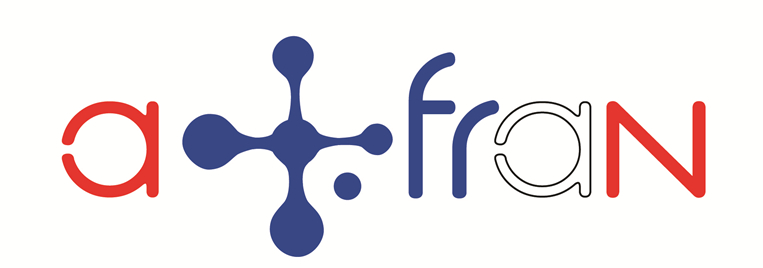
Instructor: Dr Kim-Anh Lê Cao and Dr Olivier Chapleur; Tutor: Ms Laetitia Cardonna. The travels of Olivier and Laetitia is proudly sponsored by AFRAN, the Australian-French Association for Research and Innovation.
Organized by: Melbourne Integrative Genomics, University of Melbourne
Fees for 3 days are AUD500 for RHD students, AUD900 for research non-profit organisations and AUD1500 for industry / government. The Environmental Microbiology Research Initiative EMRI (University of Melbourne) proudly sponsors registration bursaries ($225 to support some of the registration costs) to 5 RHD students enrolled at UoM. Apply at the EOI survey link below.
Registrations fees include coffee breaks, lunch, lecture notes and electronic material (slides, R code, data).
Location: Theatre 4 Alan Gilbert Building, University of Melbourne
Registration Express your interest at this survey link. As we have a limited number of participants (30), priority will be given to postgraduate students and early career researchers. EOI closes on March 11.
Contacts mixomics[ at] math.univ-toulouse.fr (for pre-requisite or content)
Prerequisite and requirements We require from the trainees a good working knowledge in R programming (e.g. handling data frame, perform simple calculations and display simple graphical outputs) to fully benefit from the workshop. Participants are requested to bring their own laptop, having installed the software RStudio http://www.rstudio.com/and the R package mixOmics (instructions will be provided prior to the training).
Outline
Day 1 & 2: methods and hands-on. The following broad topics will be covered.
A. Key methodologies in mixOmics and their variants:
- Basic processing of count data (scaling, how to handle compositional data)
- Exploration of one data set and how to estimate missing values
- Identification of a microbial signature to discriminate different treatment groups
- Integration of two data sets and identification of microbial markers
- Introduction to repeated measurements or longitudinal studies analysis
- How to deal with batch effects
- Integration of more than two data sets to identify multi omics signatures (if applicable)
- Integration of independent but related studies (if applicable)
B. Review on the graphical outputs implemented in mixOmics
- Sample plot representation
- Variable plot representation for data integration
- Other useful graphical outputs
C. Case studies and applications
Several microbiome studies will be analysed using the methods presented above.
Day 3: bring your own data. Participants will be given the opportunity to analyse their own data under the guidance and the advice of the three instructors. Participants can also work in a team. Some data sets will also be provided for those unable to bring their own data.
The following statistical concepts will be introduced: covariance and correlation, multiple linear regression, classification and prediction, cross-validation, selection of microbial markers, penalised regressions. Each methodology will be illustrated on a case study (theory and application will alternate).
Target group The course is intended for microbiologists working in the fields of bioinformatics, computational biology and applied statistics with some statistical knowledge and a good working knowledge in R. It will be particularly useful to those interested in:
- Exploring microbiome data sets.
- Selecting microbial features with methods implementing LASSO-based penalisations.
- Using graphical techniques to better visualise data.
- Understanding and/or applying multivariate projection methodologies to large data sets.
Anticipated learning outcomes After completion of this workshop, participants will be able to
- Understand fundamental principles of multivariate projection-based dimension reduction technique.
- Perform statistical integration and feature selection using recently developed multivariate methodologies.
- Apply those methods to high throughput microbiome studies, including their own studies.